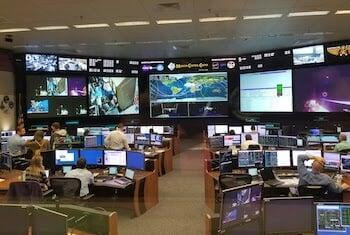
Planning process maturity & orchestration | Highlights
Planning process maturity & orchestration | Highlights
Highlights of real, practical experience from multiple discussions with supply chain practitioners from companies including:
2 Sisters Good Group, 3M, AB Sugar, AB-InBev, Abcam, adidas, AG Barr, Aggregate Industries, AkzoNobel, Aldi, Alexander Dennis, Alstom, Amcor, Amscan, Animalcare Group, AO, Apple, Arla Foods, Arysta, Asahi UK, Asics, AS Watson, ASR Group, Associated British Foods, Aston Martin, Astrak Group, Astrazeneca, Atlas Copco, Avon, Bacardi, Bakkavor, Balt Extrusion, BAT, Bausch Health, Bavaria Breweries, Beiersdorf, Belron, Berkeley Group, BMI Healthcare, Boots, BP, Bridgestone, Bristol Myers Squibb, Brita, British Sugar, Britvic, Brooks Running, BT, Bugaboo, Burberry, Burton's Biscuit Company, C&C Group, Campari, Cantel Medical, Cargill, Carlsberg, Caterpillar, Cath Kidston, Centrica, Chartlotte Tilbury, Clariant, Clarks, cmostores.com, Coca-Cola, Colorcon, Corbion, Costa Coffee, Coty, Cra'ster, Currys, Danone, Dawn Foods, Deckers, DFS, Diageo, Dr Oetker, Dreambaby, DS Smith, Dunelm, easyJet, Electrolux, Energizer, Euro Car Parts, Eurofit Group, Fairphone, Ferrero, Flo Gas, Ford, Freesat, Furniture Village, General Mills, Glory Global, Goodyear, Google, Greencore, Greggs, GRS, GSK, Hachette, Haleon, Halfords, Hallmark, Haribo, HARMAN, Hasbro, Heineken, Henkel, Hilti Corporation, Howdens, HP, HTC Europe, IBM, JCB, JDE, Jewson, John Lewis, Johnson + Johnson, Kao Corporation, Karcher, Kerry, Kimberly-Clark, KIND Snacks, Kingfisher, KP, KraftHeinz, Lactalis, LEGO, Leoni, Lululemon, LVMH, M&Co, Macmillan Education, Majestic Wine, Marks & Spencer, Mars-Wrigley, McCormick, McDonalds, Medtronic, Mondelēz, Monica Vinader, Moove Lubricants, Monsanto, Morrisons, Mountain Warehouse, Müller, Nando's, Nestlé, Nike, Novocure, Nutricia, O-I, Opple, Oriflame, Oxford University Press, Pearson, Pentland, PEP&CO, Pepsico, Pernod Ricard, Perrigo, Pfizer, Philip Morris, Philips, Pladis Global, Primark, PZ Cussons, Reckitt, Red Bull, Ricoh, River Island, RS Group, Sainsbury's, SC Johnson, Shell, Siemens Healthineers, Sky, Smith & Nephew, Sodastream, Sony, Specsavers, STADA, Starbucks, STMicro, Suntory, Superdry, Takeda, TalkTalk, Tata Consumer Goods, Tate & Lyle, Tesco, Teva, The Body Shop, The Nature's Bounty Co., ThermoFisher, The Very Group, TJX Europe, Topps Tiles, TT Electronics, Tupperware, Under Armour, Unilever, Upfield, Vision Engineering, Vivera, Vodafone, Waitrose, Walgreens Boots Alliance, Warburtons, WD-40, Westcoast, Whitworths, WHSmith, William Grant, Wickes and WLI.
Top SC Planning Best Practices...Digested
Real lessons learned distilled from a series of practitioner exchanges on...
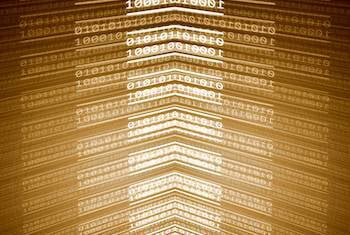
Digital Transformation of Supply Chain Planning
Digital transformation has been on corporate agendas for some time already but, borne of necessity, tangible progress accelerated significantly since 2020. A silver lining of the pandemic may be that the business case is clearer so the focus has shifted towards implementing and scaling digitalisation initiatives.
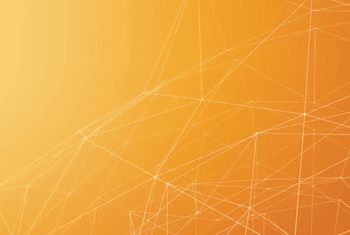
Supply Chain Design Modelling and Analytics
Supply chain network (re)design used to come around every few years or so, often based on quite a high level view of customer segments and associated costs. Now, competitiveness and even business continuity relies on the capability to dynamically adapt network flows based on a much more granular understanding of cost drivers.
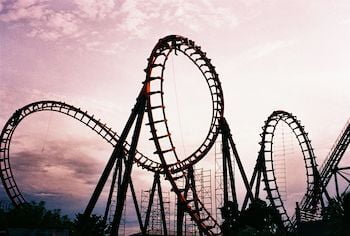
Volatility, Agility & Resilience: Next-Level Planning
Volatility, Agility & Resilience: Next-Level Planning
Volatility, uncertainty, complexity and ambiguity was already an increasing factor before the pandemic but, now, it is clear that we need the next level of demand forecasting, sensing, planning and execution.