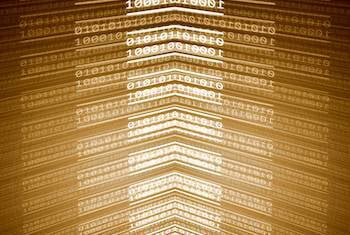
Digital Transformation of Supply Chain Planning
Digital Transformation of Supply Chain Planning
This is a combined and condensed summary of a series of best practice exchanges among supply chain directors on the digital transformation of planning. The contributions have been anonymised and generalised from their original, specific contexts to respect participants' privacy.
Planning digitalisation - big picture challenges
- Having a clear and unified understanding for what the strategic goals of supply chain and planning digitalisation are and how they support overall business strategy;
- Knowing what the realistic capabilities of digital technologies are and what is hype;
- ‘Future-proofing’ digitalisation initiatives to minimise the risks that technologies invested in become obsolete before time or are superseded by new technologies or standards;
- Whether to build proprietary, in-house capabilities or use external service providers (or some combination of the two;
- Balancing technological capabilities in harmony with process and people capabilities so that they reinforce each other rather than one or other taking precedence. This may require fundamental redesigns of job roles and core processes;
- Prioritising, coordinating and sequencing the many potential digitalisation initiatives to demonstrate RoI whilst simultaneously building confidence and buy-in at all levels. This should seek to integrate digitalisation projects into a coherent whole rather than risk disconnected and limited pilot projects scattered across different silos;
- When appropriate, choosing the right technologies and partners for successful implementation and ensuring that different systems and platforms are fully integrated;
- Change management: addressing the fear and misconceptions that often surround digitialisation programmes; implementing and embedding changes so they last once the project leaders have moved on.
Looking specifically at supply chain planning digitalisation, the main focus has been on the potential for machine learning, artificial intelligence and predictive analytics. The main use cases discussed by participating members include:
- Trend detection: statistical patterns for business KPIs;
- Anomaly detection: deviation from normal behaviour;
- Predictive capability: learning from machine data and business context;
- Prescriptive capability: recommended actions based on root cause analysis.
- The belief that AI technology is generally not mature enough yet for plug & play. In reality, it has been a mixed experience whereby some default options have been a good fit whereas some big-name products needed lots of rework;
- IT departments can be territorial about master data and often have a bias towards building in-house capability but this can make it less accessible and user friendly if not designed with a clear understanding of the end goals;
- As ever, building confidence in initiatives and getting over fear factor which sometimes needed a leap of faith and trust in the algorithm before trying to unpack There were few overnight successes and the vast majority certainly required time & effort;
- Another fear factor was that ML/AI is going to kill jobs which created passive (and, in some cases, active!) resistance. It’s vital to work with HR to clarify the intended use and impact of ML/AI and make clear it’s about augmenting people’s roles not replacing them;
- Too much and/or poor quality data: the old adage about garbage in, garbage out still holds true and even the best algorithms and models will perform poorly with low quality data. A substantial about of human input is required to guide & define this process;
- More than ever, demonstrating business cases and ROI at a time when investment capital is at a premium. Especially when around 80% of current IT budgets go on maintenance of current infrastructure;
- Planning, managing & leveraging digital transformation at an industrial scale across a global business with many moving parts.
Overcoming barriers
- Question the fundamentals of the business before making large, difficult to reverse ML/AI investments. For example, if you’re a consumer goods business, the Covid-19 experience may prompt you to consider if you may need to develop a D2C biz model? That would change the SC model from from make-to-stock to make-to-order in real time, with smaller lot sizes which would require an online presence and tech enablement for an agile SC. All of that would shape the data strategy that ML/AI would be applied to;
- Decide on your investment pipeline...how much can you afford to do? Continue to explore or pare back and revisit existing use cases to see if it’s possible to leverage / scale up what’s already been done?;
- Strategic business partnering: implanting data scientists in other functions to help build algorithms which understand and respond to the right context;
- Platform approach: look for use cases that could be deployed across business units and subsidiaries;
- Prepare stakeholders for the digital transformation journey to evolve as you travel…neither the destination nor the route should be written in stone.
Selecting a planning tool
Top recommendations from participants' experience
Carefully consider where you are at and your level of planning maturity. An audit of users is a great way to begin the process of properly understanding and formulating requirements;
Process is equally, if not more, important than the tool. Do you need / are you ready for the highest level of functionality right away?;
Resilience is now a core component of digitalisation roadmaps so how is that defined for your business? The goal should be for the tool to help planning better orchestrate the end-to-end supply chain…not just make the forecast more accurate;
Consider the 4 dimensions of people, process, data and systems when you are defining priorities and forming your roadmap; capabilities need to be developed in all 4 dimensions to achieve best outcomes. In particular, the people dimension is absolutely critical so be prepared to rethink what the ‘planner of the future’ looks like;
The level of vendors’ ongoing investment into their software is a key criteria as tools are likely to continue evolving at speed;
Agree a timeframe upfront: selection should be a 12-week process (or less). Beyond that, it can run out of steam;
Using a tool selection framework is important to structure thinking: aim to create a shortlist of max four companies; conduct a proof of concept and reference vendors by talking to other users;
Buy-in: make the selection process transparent. Though it’s vital to get the buy-in of supply chain professionals, shifting the mindset of the wider business through effective change management is central to success. Overall, transforming an organisational mentality is about taking every employee on a journey; communicating the individual value of the changes for them, as well as what it means for the business going forwards. Great technology is only as good as the people using it. If employees aren’t convinced that new solutions will benefit them or their roles, siloed thinking and use of traditional tools such as Excel will continue, even if there are better technologies available.
Key considerations
- Should I be looking at next generation systems with out-of-the-box machine learning AI capability?;
- Single vs. multiple tools? How advantageous is it to use a single vendor?;
- Make or buy? In specific contexts with particular requirements, developing in-house capability may pay off. Bear in mind that some tools offer a ‘best of both worlds’ with, for example, out-of-the-box algorithms for the majority of machine learning demands but also capability for customisation within the same tool;
- Usability: if you have an in-house or particularly complex tool and your superusers move on, you could have a major problem;
- Getting right tools for job: job needs to drive tool, not other way around;
- Too many tools, not enough integration can cause organisations to act in silos;
- Transition into various tools: supply chain-centred but accessible to other functions;
- Dashboards and simplified visualisation are helpful to buy-in and consensus;
- Can the tool choose the right forecasting model?
Securing budget
Establishing robust business cases, winning senior management as well as stakeholder buy-in and, finally, securing the budget for new investments are among the mostly hotly debated topics of all.
Supply chain professionals generally tend to appreciate the scale and nature of the transformation at hand, but how should this be best communicated at board level, particularly when there might not be many / any members with a supply chain background?
Typically, SCDs needed to make the case for SC investments at two levels – first the executive team and then, if corporate governance requires it, the board. An effective business management process such as IBP (sometimes referred to as advanced S&OP) was the main platform which consolidated the product, demand, supply chain and financial plans for the business in preparation for senior meetings where the plan’s business decisions were taken. This process helped establish that investment is essential for the delivery of the plan as signed off by all functions, making a clear case to the executive team with all the detail it needed to present the investment to the board for approval.
Without such a process, it was generally more of a challenge to clearly explain the business deliverables of the investment and show the linkage to the business strategy. A key enabler was to work with the finance team to develop the return on investment and build out the business case with the broader non-financial deliverables of the investment.
With thanks to participants from...
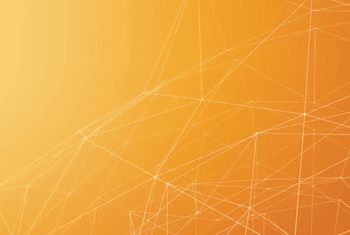
Supply Chain Design Modelling and Analytics
Supply Chain Design Modelling and Analytics
Supply chain network (re)design used to come around every few years or so, often based on quite a high level view of customer segments and associated costs. Now, competitiveness and even business continuity relies on the capability to dynamically adapt network flows based on a much more granular understanding of cost drivers.
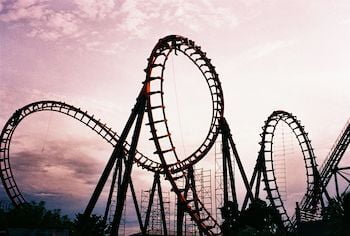
Volatility, Agility & Resilience: Next-Level Planning
Volatility, Agility & Resilience: Next-Level Planning
Volatility, uncertainty, complexity and ambiguity was already an increasing factor before the pandemic but, now, it is clear that we need the next level of demand forecasting, sensing, planning and execution.