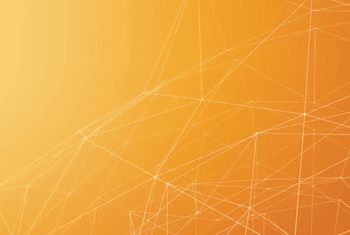
Supply Chain Design Modelling and Analytics
Supply Chain Design Modelling and Analytics
This is a combined and condensed summary of a series of best practice exchanges among supply chain directors on supply chain design, analysis & scenario modelling. The contributions have been anonymised and generalised from their original, specific contexts to respect participants' privacy.
Supply chain design & scenario modelling
How does a digital twin help with supply chain design modelling?Supply chain design involves creating a high definition digital replica of the physical supply chain which can be used to optimise performance, evaluate alternatives and determine the impact of changes in a safe, risk-free environment. This supports fast, evidence-based decision making and allows organisations to embark on strategic supply chain transformation programmes with confidence that the changes they make will deliver the expected outcomes.
Potential benefits:- Clarity: supply chains evolve over time and accumulate features that inhibit optimal performance due to acquisitions / mergers / divestments, changing strategies, customers and product portfolios, successive technologies, legacy systems etc. Supply chain design modelling introduces a discipline to step back from execution and planning mode into a design mode where default assumptions are challenged and potential new efficiencies are revealed;
- Granular visibility: by necessity, management teams focus on KPIs which tend to be high level and paint a certain picture of the business. However, they potentially over-simplify the real picture and so obscure issues and opportunities that are revealed at a more granular level;
- Risk mitigation: enables anticipation and preparation for potential shocks and disruption, opening the doors for greater agility;
- Higher quality decisions: the discipline of design forces critical evaluation and, once a model is in place, compels decisions to be evidence-based, rationalised and quantified, thereby reducing or eliminating bias. The digital twin / model also helps to democratise sophisticated modelling across business units and functions so reducing potential blind spots and leading to better, more sustainable decisions;
- Agility / faster decisions: near real-time decision-making becomes possible as the potential impacts of any proposed course of action are modelled and so don’t need to be calculated from zero;
- Enable further digitalisation: an effective model may enable use of other digital technologies such as RPA, machine learning and artificial intelligence which offer further efficiencies.
Potential challenges:- Implementation cost: as ever, it requires significant investment in terms of time, money and effort which also implies opportunity costs;
- Theory vs. practice: can be a disconnect between what is possible and desirable in the model versus what is actionable in reality;
- Continual investment: supply chain design modelling should not be a one-off or occasional exercise; it must be continually updated to incorporate changes and new data.
How is supply chain design modelling being used by discussion participants?During the pandemic it was being used reflexively, as an urgent response to potential threats to plan for continuity of business operations. As we move to a post-pandemic context, it is increasingly being used for optimisation of inventory levels, product portfolios and lead time impacts.
Use cases included:- Closing gap between plan and execution based on unified view and mutual understanding
- Post-plan analytics to determine where errors were made
- Footprint optimisation project which reduced spend by 20%
- Cost-to-serve analysis & improved customer segmentation
- Modelling and optimisation of distribution network
- Contingency / continuity planning, including related to Brexit impact
- Also done for tier 2 & 3 suppliers
- Shifted from make-to-order to make-to-stock
- Advanced analytics to improve data-driven planning & decision-making
- CO2 analysis & optimisation
- Omni-channel distribution analysis
- Supply / capacity planning
- Network design & redesign in response to supply disruptions
Best practice: Supply chain design modelling- Start small and iterate;
- Often easier to gain support for a limited project where the need and potential benefits are broadly understood
- helps build the business case for further investment in modelling and gain support from senior leaders / project sponsors;
- can mean that the model is fragmented / skewed by the narrower focus that may take more effort to amend later;
- Start with comprehensive design;
- this usually involves starting by compiling and harmonising data from multiple sources to build the “digital decision layer”;
- this approach encourages a more comprehensive, “root and branch” analysis and understanding of the supply chain which, once complete, allows the model to be applied to a much wider range of areas and challenges and so can pay bigger dividends sooner in terms of competitiveness;
- can still do projects sequentially but begin with the end in mind;
- can be a trade-off between thoroughness and agility: some decisions need to be made faster than comprehensive analysis may allow;
- Make dynamic, not static:
- the more up-to-date the model is - for example, with live material prices, currencies etc. - the more effective the analysis and decisions that emerge from it;
- this requires a more fundamental shift in mindsets and requires more stakeholder buy-in;
- Winning hearts and minds: decisions from models can sometimes challenge the intuition of experienced team members so effective representation of decisions is vital.
- Clarity: supply chains evolve over time and accumulate features that inhibit optimal performance due to acquisitions / mergers / divestments, changing strategies, customers and product portfolios, successive technologies, legacy systems etc. Supply chain design modelling introduces a discipline to step back from execution and planning mode into a design mode where default assumptions are challenged and potential new efficiencies are revealed;
- Granular visibility: by necessity, management teams focus on KPIs which tend to be high level and paint a certain picture of the business. However, they potentially over-simplify the real picture and so obscure issues and opportunities that are revealed at a more granular level;
- Risk mitigation: enables anticipation and preparation for potential shocks and disruption, opening the doors for greater agility;
- Higher quality decisions: the discipline of design forces critical evaluation and, once a model is in place, compels decisions to be evidence-based, rationalised and quantified, thereby reducing or eliminating bias. The digital twin / model also helps to democratise sophisticated modelling across business units and functions so reducing potential blind spots and leading to better, more sustainable decisions;
- Agility / faster decisions: near real-time decision-making becomes possible as the potential impacts of any proposed course of action are modelled and so don’t need to be calculated from zero;
- Enable further digitalisation: an effective model may enable use of other digital technologies such as RPA, machine learning and artificial intelligence which offer further efficiencies.
- Implementation cost: as ever, it requires significant investment in terms of time, money and effort which also implies opportunity costs;
- Theory vs. practice: can be a disconnect between what is possible and desirable in the model versus what is actionable in reality;
- Continual investment: supply chain design modelling should not be a one-off or occasional exercise; it must be continually updated to incorporate changes and new data.
- Closing gap between plan and execution based on unified view and mutual understanding
- Post-plan analytics to determine where errors were made
- Footprint optimisation project which reduced spend by 20%
- Cost-to-serve analysis & improved customer segmentation
- Modelling and optimisation of distribution network
- Contingency / continuity planning, including related to Brexit impact
- Also done for tier 2 & 3 suppliers
- Shifted from make-to-order to make-to-stock
- Advanced analytics to improve data-driven planning & decision-making
- CO2 analysis & optimisation
- Omni-channel distribution analysis
- Supply / capacity planning
- Network design & redesign in response to supply disruptions
- Start small and iterate;
- Often easier to gain support for a limited project where the need and potential benefits are broadly understood
- helps build the business case for further investment in modelling and gain support from senior leaders / project sponsors;
- can mean that the model is fragmented / skewed by the narrower focus that may take more effort to amend later;
- Start with comprehensive design;
- this usually involves starting by compiling and harmonising data from multiple sources to build the “digital decision layer”;
- this approach encourages a more comprehensive, “root and branch” analysis and understanding of the supply chain which, once complete, allows the model to be applied to a much wider range of areas and challenges and so can pay bigger dividends sooner in terms of competitiveness;
- can still do projects sequentially but begin with the end in mind;
- can be a trade-off between thoroughness and agility: some decisions need to be made faster than comprehensive analysis may allow;
- Make dynamic, not static:
- the more up-to-date the model is - for example, with live material prices, currencies etc. - the more effective the analysis and decisions that emerge from it;
- this requires a more fundamental shift in mindsets and requires more stakeholder buy-in;
- Winning hearts and minds: decisions from models can sometimes challenge the intuition of experienced team members so effective representation of decisions is vital.
Cost-to-Serve (CTS) analytics
- When data is available, it's not necessarily complete or transparent and the results are sub-optimal, where a conclusion isn't always obvious;
- CTS is less commonly used to drive routine discussions and decision-making between functions;
- CTS does not always drive pricing to customer - yet it can and perhaps should;
- Looking at CTS further up the supply chain quickly becomes complex;
- Without data in a usable form readily at hand, the situation has often already changed by the time an analysis can be done;
- One-off CTS analyses typically only provide a snapshot which, while interesting and possibly informative, rarely becomes actionable as an ongoing tool which limits their practical value;
- As greater emphasis is placed on supply chain resilience, there is greater need to be able to model the implications of possible configurations of footprints, supplier terms etc. and understand it's impact on the bottom line.
- Identifying the strategic SKUs: new SKUs, range credibility SKUs or those that prevent competitors from getting a foothold in the market – these should be retained, regularly reviewed with a view to improving profit;
- Profit improvement: can we identify actions and interventions at specific points in the supply chain to improve SKU profitability e.g. changes to fulfilment channels / routes and controls around order multiples;
- SKUs that cannot be made profitable and are not strategic are the real candidates for range reduction…the critical element is having the right information to get to this point
- COST-to-serve is somewhat of a misnomer as it can overly narrow the focus on the cost side whereas framing it as something like 'cost-to-serve contribution', 'Holistic Customer Investment' or taking an EBIT-level view offers better perspective coupled with and end-to-end view;
- Incorporate data from other parts of the business in whatever format it is recorded (don’t try to introduce or change data format as this will surely be impeded);
- Start with the invoice data as a base and go to each function in your organisation to see what data can be pulled to augment this?;
- Set the CTS rules and get buy-in to allocation methodology. The warehouse is often the most complex area;
- Ensure CTS is not being used to ‘beat up’ one function. Transparency of CTS data helps defuse a sometimes adversarial dynamic where one function or teams' view is pitted against another, acting as a glue rather than a wedge. This transparency itself often prompts more and better questions which are enriched by different viewpoints;
- Factor in FTE overhead e.g. how many planners, how much devotion in customer service? Those FTEs are most often allocated to customers, or geo’s, or products, so these costs can be attributed;
- Establish the wins for each function and you’ll get the buy-in! How does better CTS insight help each function perform its role better and contribute to the common goal e.g. improving EBIT? Consider extending the potential “wins” to include suppliers who may need to be motivated to support upstream enhancements (open book discussions). This could be particularly significant in the ESG expectations of suppliers;
- CTS can start in supply chain but often end up in commercial where it is used as a framework for productive negotiation and to drive deals and offers. Sharing this information with customers can help influence how they accept being served, or nudge buying practices in a positive way;
- Make CTS a living breathing exercise - take data feeds once a month. A one-off exercise tends to land and be ignored because there is no change or measurement for improvement. CTS analysis can then be used as a data feed for other changes, projects or servicing decisions.
- CTS initiatives have been broadly successful and been worth the investment;
- CTS means different things to different people so important to be clear: it’s more than cost-to-fulfil and should incorporate gross price and margin;
- Complex interdependencies means that brand-level analysis is too coarse and misses the vital interdependencies between behaviours and cost drivers;
- Specify & validate basic assumptions about core drivers of cost in the network and then focus on just a few SKUs that hold the most potential savings as there’s too much complexity to be comprehensive;
- focus on the main cost drivers and allocate general ledger costs on a volume or value basis;
- important to combine at customer level;
- also consider the cash position: two customers may look the same but, once their payment history and a cost of capital is factored in, it can make a 15-20% difference in their profitability;
- An 80:20 pattern is common where 80% of the profitability is generated by 20% of the SKUs
- cutting SKU tails may mean pushing some customers to be served through wholesalers rather than direct
- have to be careful: even unprofitable SKUs may be contributing to fixed asset costs. Cutting them leads to cost absorption for other SKUs...the volume needs to be replaced with more profitable SKUs
- Defining costs is important but isn’t the ultimate goal...even if the cost can’t be precisely quantified, there is still high confidence that a course of action will save money so shouldn’t be delayed just because a precise saving hasn’t yet been calculated
- General trade terms can offer a better lever to improve CTS rather than individual customer terms which become too complex to monitor and manager
- can’t expect to cut penalties entirely but a few classes of trade terms help set better frameworks & KPIs for managing CTS
- this may mean the same customer in different classes if, for example, they have different internal customer e.g. convenience stores compared to supermarkets
- can include terms that help data capture
- terms can be leveraged with a B2B customer portal by incentivising more profitable behaviours
Ongoing challenges:
- Data integration: overlay / integration with ERP or incorporating data from 3PLs? Especially secondary freight can be a black box
- Collaborating with commercial teams who are reluctant to risk relationships by talking about perceived constraints to service. This is where a projected saving is helpful to aid the rationale for such customer conversations.
- Often KPIs on NPD which can result in 100 new SKUs instead of just the best 10
With thanks to participants from...
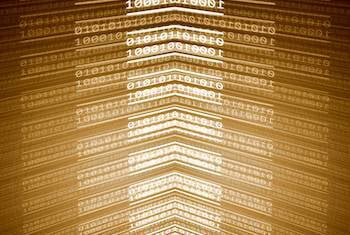
Digital Transformation of Supply Chain Planning
Digital transformation has been on corporate agendas for some time already but, borne of necessity, tangible progress accelerated significantly since 2020. A silver lining of the pandemic may be that the business case is clearer so the focus has shifted towards implementing and scaling digitalisation initiatives.
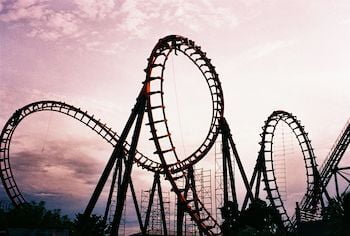
Volatility, Agility & Resilience: Next-Level Planning
Volatility, Agility & Resilience: Next-Level Planning
Volatility, uncertainty, complexity and ambiguity was already an increasing factor before the pandemic but, now, it is clear that we need the next level of demand forecasting, sensing, planning and execution.